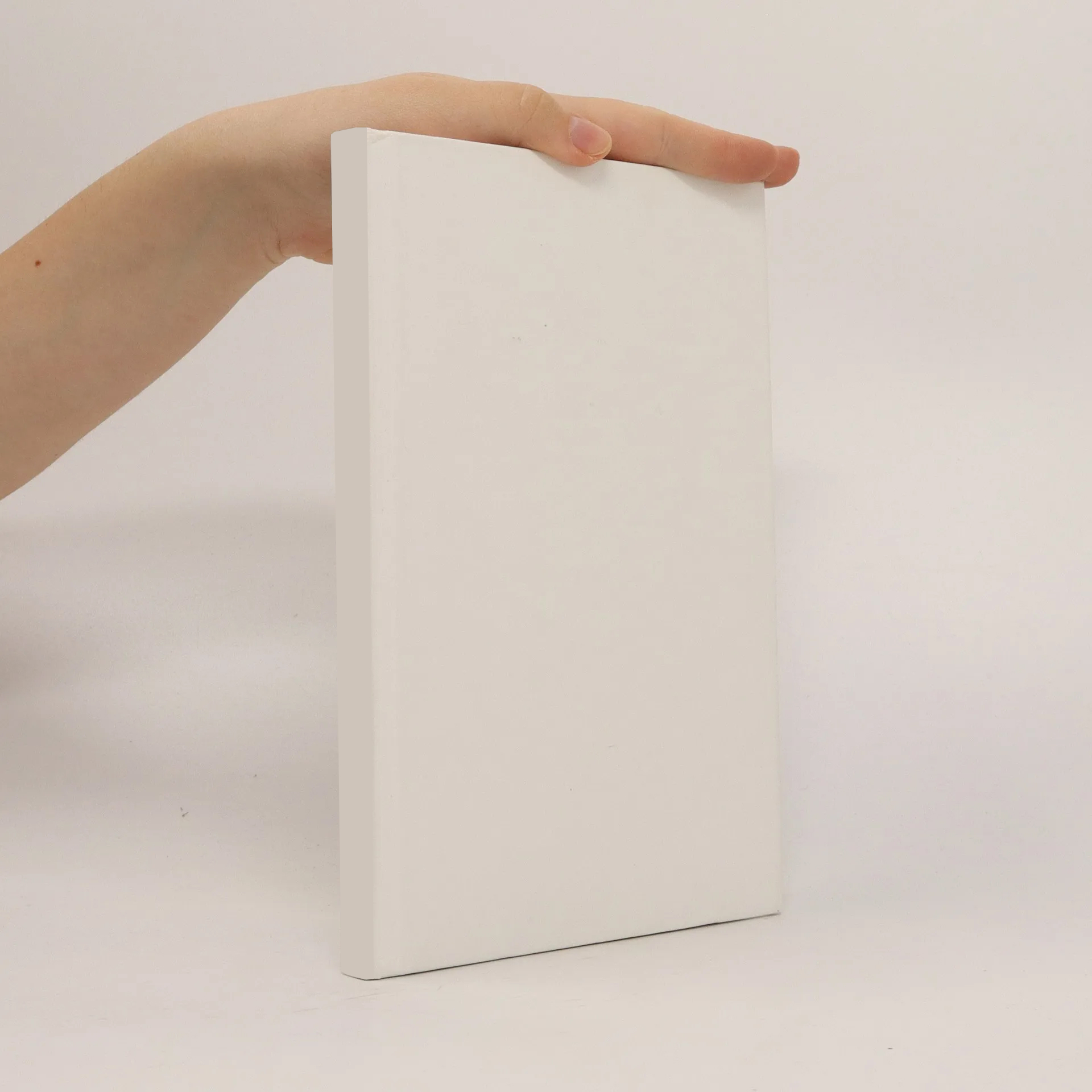
Intelligent single-channel methods for multi-source audio analysis
Autoren
Mehr zum Buch
This thesis investigates the potential of recent machine learning methods for the challenging task of single-channel, multi-source audio audio analysis, i. e., information extraction from single-channel audio where the sources of interest (e. g., speech) are mixed with multiple interfering sources. First, it is shown that source separation by recently proposed techniques for non-negative matrix factorization can significantly improve the recognition performance, compared to the state-of-the-art approach of training the recognition task with multi-source data. Second, it is shown that by formulating the source separation problem itself as a recognition task, state-of-the-art methods for supervised training of recognition systems such as deep neural network models can be used to achieve previously unseen performance in single-channel source separation. In this context, supervised training of non-negative models is introduced as well. The task of multi-source recognition as defined above is exemplified by challenging real-world speech separation and recognition problems where speech is mixed with non-stationary background noise such as music, and world-leading results in international evaluation campaigns are demonstrated for this task. Furthermore, state-of-the-art results are presented in selected music information retrieval applications involving polyphonic audio, such as characterizing the singer, or transcribing the music into a score.